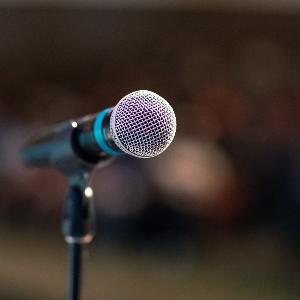
© pixabay
Title: How to "Improve" Prediction Using Behavior Modification
About the Talk: Many internet platforms that collect behavioral big data use it to predict user behavior for internal purposes and for their business customers (e.g., advertisers, insurers, security forces, governments, political consulting firms) who utilize the predictions for personalization, targeting, and other decision-making. Improving predictive accuracy is therefore extremely valuable. Data science researchers design algorithms, models, and approaches to improve prediction. Prediction is also improved with larger and richer data. Beyond improving algorithms and data, platforms can stealthily achieve better prediction accuracy by "pushing" users' behaviors towards their predicted values, using behavior modification techniques, thereby demonstrating more certain predictions. Such apparent "improved" prediction can unintentionally result from employing reinforcement learning algorithms that combine prediction and behavior modification. This strategy is absent from the machine learning and statistics literature. Investigating its properties requires integrating causal with predictive notation. To this end, we incorporate Pearl's causal do(.) operator into the predictive vocabulary. We then decompose the expected prediction error given behavior modification, and identify the components impacting predictive power. Our derivation elucidates implications of such behavior modification to data scientists, platforms, their customers, and the humans whose behavior is manipulated. Behavior modification can make users' behavior more predictable and even more homogeneous; yet this apparent predictability might not generalize when customers use predictions in practice. Outcomes pushed towards their predictions can be at odds with customers' intentions, and harmful to manipulated users.
About the Speaker: Galit Shmueli is the Tsing Hua Chair Professor at the Institute of Service Science, College of Technology Management, National Tsing Hua University, Taiwan. Earlier she was Associate Professor at University of Maryland's Smith School of Business, and then the SRITNE Chaired Professor of Data Analytics and Associate Professor of Statistics & Information Systems at the Indian School of Business. Prof. Shmueli’s research focuses on statistical and machine learning methodology with applications in information systems and healthcare, and an emphasis on human behavior. Since her 2010 Statistical Science paper “To Explain or To Predict?” (2000+ citations), she's been investigating how predictive methodology can enhance causal explanatory goals, and how causal explanatory methodology can enhance predictive goals. Prof. Shmueli authors multiple books, including the popular textbook Machine Learning for Business Analytics and has over 100 publications in peer-reviewed journals and books. Prof. Shmueli teaches courses on machine learning, forecasting analytics, interactive visualization, research methods, and other business analytics topics. Her online teaching videos are highly subscribed, and she has won multiple teaching awards. Prof. Shmueli was the inaugural Editor-in-Chief of the INFORMS Journal on Data Science (2020-2024), and has served on editorial boards of top journals in statistics and information systems. She is an INFORMS Information Systems Society (ISS) Distinguished Fellow, an Institute of Mathematical Statistics (IMS) Fellow, and International Statistical Institute (ISI) Elected Member.