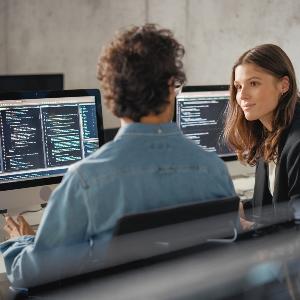
Teaching the next generation of AI experts
The chair of AI in Management is engaged in developing, implementing and evaluating AI to improve management. This is also what we try to deliver to students through our teaching. Our focus is on AI algorithms and coding.
Skip to content
Courses
Courses in Summer Semester
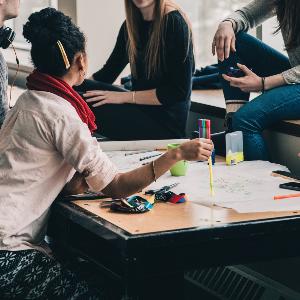
© pixabay
All courses and assessments are in English!
Master
- AI for Good (Seminar)
- Managerial AI (Seminar)
- Methods for AI (Seminar)
- Business Analytics in Practice (Projektkurs)
Bachelor
- AI Tools for Management and Social Science (Hauptseminar)
Course Design
In line with our digital focus, we apply a digital and innovative teaching approach and course design. All materials and interactions are coordinated via Moodle. Courses and assessments are in English. We practice an annual rotation of courses, with the exception of the Hauptseminar, which takes place every semester.
Course Sequence
For our Master's courses, we recommend the following sequence (1) AI for Managers [MMT & MBR] or Digital Technologies, Business Analytics and Management [M.Sc. BWL] => (2) Advanced AI in Businesses and Organizations => (3) Research in AI and Management.
Courses in Winter Semester
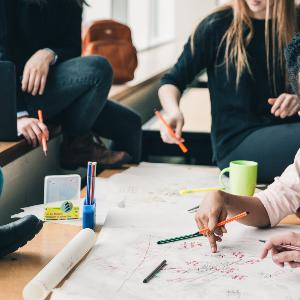
© pixabay
All courses and assessments are in English!
Master
- AI for Managers (Lecture)
- Advanced AI in Businesses and Organiztions (Seminar)
- Digital Technologies, Business Analytics and Management (Fachspezifische Grundlagen, FSG)
Bachelor
- Introduction to AI (Lecture)
- AI Tools for Management and Social Science (Hauptseminar)
Course Design
In line with our digital focus, we apply a digital and innovative teaching approach and course design. All materials and interactions are coordinated via Moodle. Courses and assessments are in English. We practice an annual rotation of courses, with the exception of the Hauptseminar, which takes place every semester.
Course Sequence
For our Master's courses, we recommend the following sequence (1) AI for Managers [MMT & MBR] or Digital Technologies, Business Analytics and Management [M.Sc. BWL] => (2) Advanced AI in Businesses and Organizations => (3) Research in AI and Management.
LSF and Moodle
All our courses are listed in LSF. We ask for self-enrolment via Moodle. The self-enrolment keys are available via LSF. Please find all relevant information about the courses on our Moodle course pages. We recommend reading all information there carefully. The teaching language is English.
Theses
M.Sc. Thesis
We offer inovative and impactful topics (e.g. AI for Good, AI for Medicine, Causal Machine Learning, etc.) that we jointly select to fit the interests and study focus of students. Due to the double-affiliation to the department of Mathematics, Informatics and Statistics, we also welcome students from there to apply for a Master thesis at our chair.
Requirements
- For business students:
- Substantial programming skills
- Prior participation in our courses: (1) AI for Managers [MMT] or Digital Technologies, Business Analytics and Management (FSG) [M.Sc. BWL]; and (2) Advanced AI in Businesses and Organizations (Highly recommended)
- For students in mathematics, informatics and statistics: No requirements
Specifications
- English language
- LaTeX format
- Topics are provided by the chair
- Industry-based theses are possible, but must be organized by students themselves (please follow the same application procedure as for regular theses below)
Application
- Application via email ai@som.lmu.de
- Provide the following documents and information: (1) Curriculum Vitae, (2) transcripts (Master & Bachelor), and (3) preferred start date
- You can also state our interestes / preference of thesis topics => Please check the tab "Topics"
Colloquium
- To support research-oriented focus of the theses, we regularly hold a keynote series around "AI in management". Thesis students are expected to participate.
Tutorial
Download our thesis tutorial (PDF, 179 KB)
B.Sc. Thesis
We offer inovative and impactful topics (e.g. AI for Good, AI for Medicine, Causal Machine Learning, etc.) that we jointly select to fit the interests and study focus of students. Due to the double-affiliation to the department of Mathematics, Informatics and Statistics, we also welcome students from there to apply for a Bachelor thesis at our chair.
Requirements
- For business students:
- Basic programming skills
- Prior participation in our course Introduction to AI (Highly recommended)
- For students in mathematics, informatics and statistics: No requirements
Specifications
- English language
- LaTeX format
- Topics are provided by the chair
- Industry based theses possible, but must be organized by students themselves
Application
- Application via email ai@som.lmu.de
- Provide the following documents and information (1) Curriculum Vitae, (2) transcript, (3) preferred start date
Colloquium
- To support research-oriented focus of the theses, we regularly hold a keynote series around "AI in management". Thesis students are expected to participate.
Tutorial
Download our thesis tutorial (PDF, 179 KB)
Thesis Topics and Examples
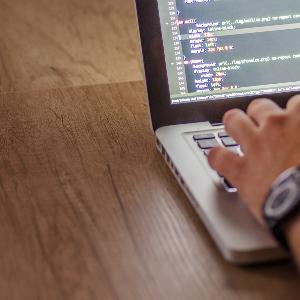
© Lukas via pexels
This is a list of current topics and broader research areas we are interested in. They apply to both Bachelor and Master theses.
Social Media Analytics
- Monitoring political ads on social media (data preparation)
- Understanding the role of social media ads in election outcomes (regression analysis)
- Identifying and mitigating toxic content (e.g., fake news, hate, speech, propaganda) on social media with machine learning (regression analysis / machine learning)
Human-AI Collaboration
- Understanding the perception of users towards generative AI (survey/field experiment)
Business Analytics
- Understanding the innovation potential of generative AI (survey/field experiment)
- Optimal budgeting for marketing campaigns (advanced machine learning)
AI for Good
- Understanding the success of power plants in Africa (regression analysis)
- Monitoring aid development for healthcare (statistical analysis)
- Applied causal machine learning for treatment effect estimation in intensive care units
Causal Machine Learning
[Faculty of Mathematics, Informatics and Statistics only]
- Causal representation learning for treatment effects in medicine
- Causal sensitivity analysis
- Reliable off-policy learning for treatment decision-making
Examples of successful, previous theses at our Institute
- Monitoring global development aid with machine learning
- Negativity drives online news consumption
- Cascade-LSTM: A tree-structured neural classifier for detecting misinformation cascades
- AttDMM: An Attentive Deep Markov Model for Risk Scoring in Intensive Care Unit
- Learning optimal dynamic treatment regimes using causal tree methods in medicine
References
We are happy to provide students of our chair with a reference letter, if they fulfill the following requirements: (1) Prior participation in our courses, (2) Successful completion of the exams. Please contact ai@som.lmu.de with your request.
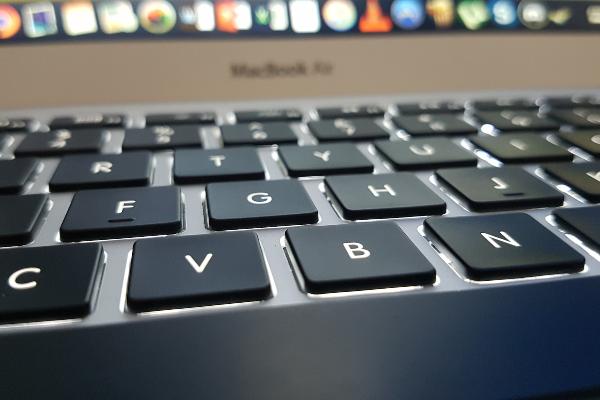