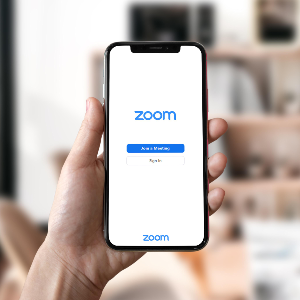
© pixabay
Wir, das Institute of AI in Management an der LMU München, sind begeistert von KI und den dynamischen Entwicklungen in diesem Bereich. Deshalb möchten wir Einblicke aus erster Hand in die aktuelle Forschungsarbeit von angesehenen Wissenschaftler:innen aus der ganzen Welt, geben. Wir freuen uns jedes Semester großartige Gastredner:innen für unsere Vortragsreihe gewinnen zu können.
Alle Vorträge finden online statt und sind per Zoom erreichbar für jeden, der Interesse hat. Unser Ziel ist es einen Überblick über aktuelle Trends in der KI Forschung zu geben. Die Vorträge finden immer am Donnerstag statt und bestehen aus ca. 45-60 Minuten Präsentation, gefolgt von Diskussion, Feedback und Q&A. Wir freuen uns darauf, Sie herzlich begrüßen zu dürfen.
Alle Informationen zu Terminen, Gastredner:innen und ihren Themen, inkl. Zoom Links werden im Laufe der Zeit auf dieser Veranstaltungsseite veröffentlicht.
Sie sind herzlich eingeladen sich für unseren Newsletter anzumelden, über den wir alle kommenden Veranstaltungen dieser Serie kommunizieren. Hier geht es zu unserer Anmeldeseite.
Die Terminserie ist eine gemeinsame Initiative mit Partnern von führenden nationalen Universitäten, unter der Leitung von Prof. Stefan Feuerriegel, LMU München:
- Prof. Markus Weinmann, University of Cologne
- Prof. Stefan Lessmann, Humboldt University Berlin
- Prof. Mathias Kraus, Friedrich-Alexander University Erlangen-Nuremberg
- Prof. Niklas Kühl, University of Bayreuth
- Dr. Michael Vössing, Karlsruhe Institute of Technology
- Prof. Oliver Müller, University of Paderborn
- Prof. Nicolas Pröllochs, Justus-Liebig-University Gießen
- Prof. Christian Janiesch, TU Dortmund
- Prof. Gunther Gust, University of Würzburg
- Prof. Tobias Brandt, University of Münster
- Prof. Yash Raj Shrestha, University of Lausanne
- Prof. Burkhardt Funk, Leuphana University Lüneburg
- Prof. Nadja Klein, TU Dortmund
- Prof. Martin Spindler, University of Hamburg
- Prof. Niki Kilbertus, TU Munich
- Prof. Stefan Bauer, TU Munich
- Prof. Henner Gimpel, University of Hohenheim
- Prof. Alexander Benlian, TU Darmstadt
- Prof. Oliver Hinz, Goethe University, Frankfurt
- Prof. Ekaterina Jussupow, TU Darmstadt
- Prof. Anne-Sophie Mayer, Vrije Universiteit Amsterdam
Do. 20.03.2025
Gastredner: Alejandro Schuler, Division of Biostatistics, UC Berkeley
Präsentation: RieszBoost: Gradient Boosting for Riesz Regression
Do. 13.02.2025
Gastredner: Alex Luedtke, Department of Statistics, University of Washington
Präsentation: Simplifying Debiased Inference via Automatic Differentiation and Probabilistic Programming
Do. 09.01.2025
Gastredner: Chengchun Shi, Department of Statistics, London School of Economics and Political Science
Präsentation: Experimental Designs for A/B Testing in Marketplaces
Do. 12.12.2024
Gastredner: Mats Julius Stensrud, Chair of Biostatistics, Swiss Federal Institute of Technology Lausanne
Präsentation: On Optimal Treatment Regimes Assisted by Algorithms
Do. 28.11.2024
Gastredner: Manoel Horta Ribeiro, Department of Computer Science, Princeton University (incoming)
Präsentation: Content Curation in Online Platforms